Lowering the OOOM Impact with Text Analytics by John Ford July 17, 2017 - Blogs on Text Analytics
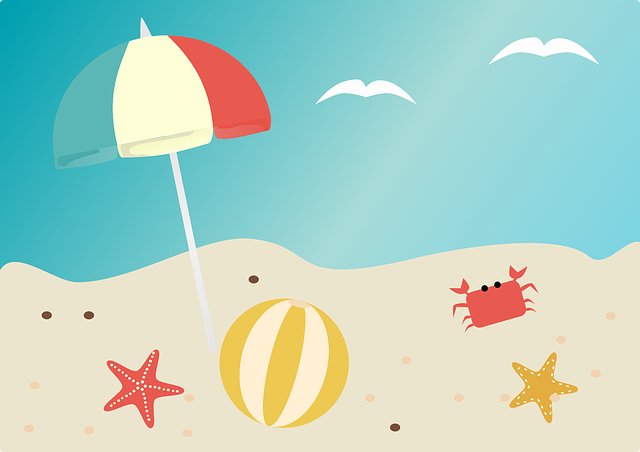
John Ford is a public sector Research Psychologist who has authored numerous reports and articles about assessment, training, and other personnel management issues. He wrote this guest blog about a recent challenge of how to deal more efficiently with out of office messages with respect to tabulating the survey response rate. In this blog he describes the techniques he used. The process may help you in your research or perhaps spark some ideas about how to solve similar issues. If you have a technique, experience, revelation to share in a blog, we would like to post it.
I work for a public-sector organization that conducts periodic large-scale surveys of government employees. We recently had a behind-the-scenes task to adjust the survey refusal rate considering those who never saw the invitation email. Text analytics helped us accomplish this task more efficiently than a low- or no-tech process would have done. We summarize our approach below and hope that the insights obtained will have value for other survey researchers.
The Problem
Initial email invitations and reminders to participate in a recent web-based survey resulted in numerous Out of Office messages (OOOMs) from individuals in our survey sample. Email account owners can create these messages and toggle their systems to return one to each sender of an incoming email. OOOMs differ from the bounced email notifications a server sends when an email address is invalid. Bounced emails are clear indications a potential survey participant has not seen the email—and did not actually refuse to take the survey.
OOOMs can be harder to classify. They are created for a variety of reasons that may or may not mean users have seen a survey invitation. Fortunately, the focused content of OOOMs makes them easier to analyze than more free-form text. OOOMs are essentially brief form letters intended to quickly and clearly communicate a specific message.
Our task was to distinguish between OOOMs indicating an employee’s absence was Temporary from those indicating a long-term or Permanent absence. Reasons for temporary absences are often unspecified, but may include short-term illness, leave (vacation), and off-site meetings. Reasons for permanent absences include resigning, being fired, retirement, surgery and recuperation, and military deployment. Accurate classification into these two categories allows the survey researchers to subtract the permanent absence count from the survey refusal rate and more accurately determine the response rate for the survey.
The Approach
The OOOM Outlook database was imported into the WordStat software from Provalis Research (Peladeau, 2017b). WordStat was configured to run without any previously constructed categorization dictionary, without an exclusion dictionary to remove unwanted “stop” words, and with lemmatization and stemming turned off so there would be no reduction of plurals and other word morphology to standard root forms of each word. This project required close examination of the unmodified language used by the OOOM authors.
Identify terms. WordStat identified the frequently-occurring words and phrases in the OOOM collection. For each term, we examined all occurrences using WordStat’s concordance (Key-Word-in-Context) feature, which enables examination of each term and the language around it. This makes it possible to quickly determine what terms mean and whether they can be used to classify. Terms that were clear and consistent indicators of either temporary or permanent absences were added to the appropriate Temporary Absence or Permanent Absence category in an OOOMs dictionary created for this project.
This process resulted in 957 terms (words and phrases) that were associated with Temporary Absence and 72 terms associated with Permanent Absence. Selected Temporary Absence and Permanent Absence themes are described in the two tables below. The different number of themes in the two categories likely reflects both the greater number of temporary OOOMs in this data set and the more direct nature of the permanent OOOM language.
Classify OOOMs. This two-category WordStat dictionary was used to classify each OOOM into one of the two absence categories. OOOMs with either no dictionary hits or hits in both categories were classified manually. Only 3,059 (13%) OOOMs needed some form of additional manual review, significantly reducing the effort required to classify the full set of OOOMs.
Temporary Absence Themes |
Example Terms |
1. The term absence is consistently used for a finite period that will end with the employee’s return. | absence, absence_from_the_office |
2. The access to or checking emails is only mentioned if the employee has ongoing responsibility to respond on behalf of the employing organization. | access_during_this_time, access_to_emails, access_to_my_email, checking_emails, email_access |
3. Identification of someone who is temporarily acting in the employee’s position only occurs if the employee will return to resume job responsibilities. | act_on_my_behalf, acting_director |
4. Specificity about the employee’s schedule below the day level only occurs when short periods of temporary absence are being described. | afternoon, end_of_the_day, evenings, monday_morning, rest_of_the_day, thursday_afternoon |
5. Some terms either directly reference or indirectly imply an eventual return to the office. | away_from_the_office_and_will_return, am_back_in_the_office, expect_to_return |
6. Emphasis of what the employee is currently or presently doing implies that this is a condition which will change in the near future. | currenlty, time frame, am_not_in_the_office_at_this_time, am_presently_out_of_the_office |
7. References to employee being in or returning to the office indicate that this will happen soon. | await_my_return, back_in_the_office, back_monday, returning, plan_to_return_to_the_office |
8. Instructions about what to do or who to contact in an emergency imply that the normal procedure is to wait for the employee’s expected return. | emergency_assistance, immediate, immediate_concerns, immediate_help, pressing_matter, urgent |
9. Terms which indicate that something (usually checking messages) will occur occasionally over a period of time signal ongoing responsibility and eventual return. | infrequent, infrequently, intermittently, limited_access, mail_during_this_time, periodically, occasionally, regularly_checking, sporadically, temporarily |
10. Direct references to leave or vacation indicate temporary absence. | leave_beginning_monday, leave_from_friday, leave_the_week_of_august |
11. References to a holiday indicate short-term leave. | labor_day |
Permanent Absence Themes | Example Terms |
1. Indicates that employee has retired. | retired_effective, am_retired, retiring |
2. Indicates that employee is no longer working there—a reason may or may not be given. | accepted_a_position, i_have_left, i_am_no_longer_with, leaving_my_position, my_last_day, no_longer_working |
3. Employee is on an outside work assignment for an extended period. | disaster_deployment, extended_deployment, i_am_currently_on_rotation |
4. Employee is on leave for an extended period. | am_out_of_the_office_on_maternity_leave, extended_leave, indefinitely, medical_leave, post_surgery_recovery |
5. A direct indication that email will not be seen. Yes, a few times it was really this simple. | can_no_longer_be_reached_through_this_email |
The Outcome
Our text analytics-enhanced review identified 710 of the OOOMs as permanent absences. This number was removed from the refusal rate for the survey, improving the rate by .3%. While not a large gain, the increase in reporting accuracy did contribute to the project. The 12 hours spent accomplishing this task was a better time investment than the many more hours of low-tech review that would have been necessary to achieve the same result, perhaps with less accuracy.
Some Thoughts
While the processes used in this project were appropriate for our behind-the-scenes classification task, the classification dictionary could have been further developed to identify more than 87% of the OOOMs. This was not a priority because the reduction of manual review to 13% of the OOOMs was a sufficient outcome and it was not clear that further development would have taken less time than review of the remaining OOOMs. Had development continued, use of rules and word patterns would have been the likely next step in this further development.
The dictionary itself is unlikely to be directly useful to other survey researchers. While OOOMs are similar across organizational settings, there is variability in the specifics of OOOM language. For example, language in this sample was noticeably influenced by the government work context, the time of year the survey was fielded, and by the military culture in some parts of the surveyed workforce.
Themes. What may be more useful to researchers adopting this approach are the Temporary and Permanent category themes identified in the two tables above. Sets of terms associated with retirement, accepting another job, and medical absences are likely to be similar in other contexts. Terms associated with absence, periodic message checking, transfer of authority, and vacation may differ somewhat, but these themes seem likely to be present in other collections of OOOMs.
Researchers should also watch for additional themes to emerge or increase in importance in other survey contexts. Different timing of this survey, for example, would likely have resulted in a different set of holiday terms and required a somewhat different strategy for interpreting them. An impending budget-driven government shutdown or other economic concerns would likely produce additional absence-related themes in workforce OOOMs. The themes from this project should be considered a useful guide, rather than a complete map of the term space for similar future projects.
Leave. A few armchair linguistics observations seem appropriate. The most interesting term in the OOOM collection is leave, along with its variations in form and context. Unlike absence, which reliably indicates a short period out of the office, the meaning of leave varies with context. By itself, it can also indicate short-term absence. But it can indicate long-term absence if it is “extended” or part of phrases like “I am leaving” or “I have left.” This highlights the importance of turning off lemmatization and other word transformations for this type of text mining task to accurately capture situations where differences in word form signal important differences in meaning.
The leave example also reinforces Tom Reamy’s (2017) repeated emphasis of the importance of context in text analytics and Normand Peladeau’s (2017b) recommendation of phrase analysis as a key component of WordStat text mining projects. This analysis also found many straightforward, word-level classification features. But the words by themselves aren’t everything—we must be crafty feature engineers (Zeng, 2017) beyond the word level to harvest full value from our deep oceans of seemingly-unfathomable text.
John Ford is a Public Sector Research Psychologist. He can be reached by email at
References
Peladeau, N. (2017a). How to build categorization dictionaries with WordStat. Webinar retrieved from https://provalisresearch.com/resources/tutorials/webinar-content-analysis-text-mining/ on 6/12/2017.
Peladeau, N. (2017b). WordStat 7.1.17. Software retrieved from https://provalisresearch.com/Download/wordstat.php on 6/12/2017.
Reamy, T. (2017). Deep text: Using text analytics to conquer information overload, get real value from social media, and add big text to big data. Information Today, Inc.: Medford, NJ.
Zeng, A. (2017). Mastering feature engineering: Principles and techniques for data scientists. O’Reilly Media: Sebastopol, CA.