AUTOMATED CLASSIFICATION OF PATIENTS FEEDBACK
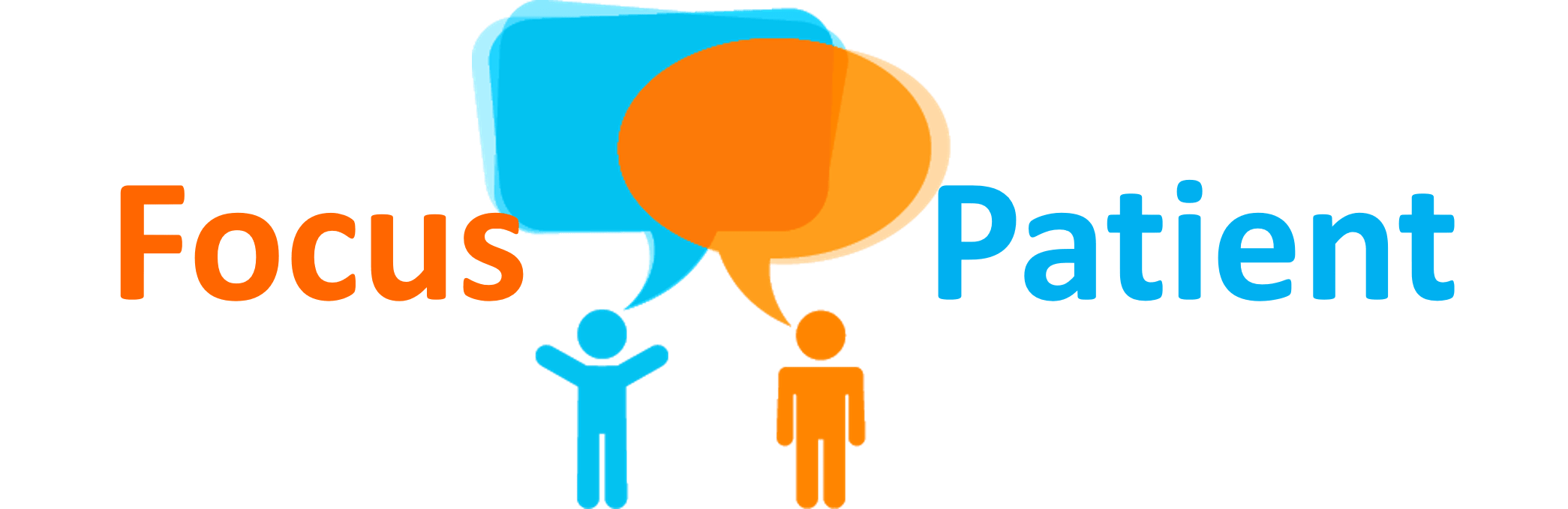
About the company
Focus Patient is a healthcare-focused consulting firm, the specialist company for Patients’ Behavior. They harvest patients’ comments by the millions from blogs, social media, and other forums. They perform an in-depth, AI-based analysis of their experiences, behaviors, and emotions. They uncover many things they do not share with their physicians which can be pivotal in providing them with optimal support and help to ensure success for our healthcare clients.
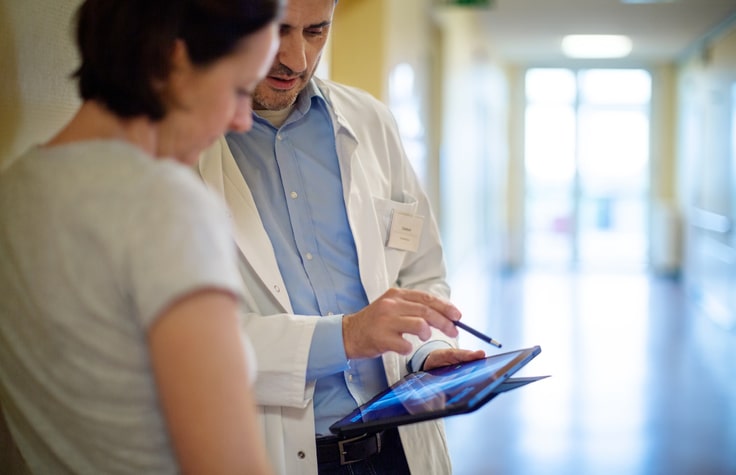
The Challenge
To process a large volume of patient comments on forums and blogs, starting with more than 35 million for France alone, Focus Patient had to individually extract themes to populate their proprietary hierarchical ontology. Going with SaaS solutions was too expensive and would not provide them with the level of control they wanted and needed. On the other hand, they did not want to build and maintain a large-scale computer infrastructure. They wanted fast, reliable, and affordable processes.
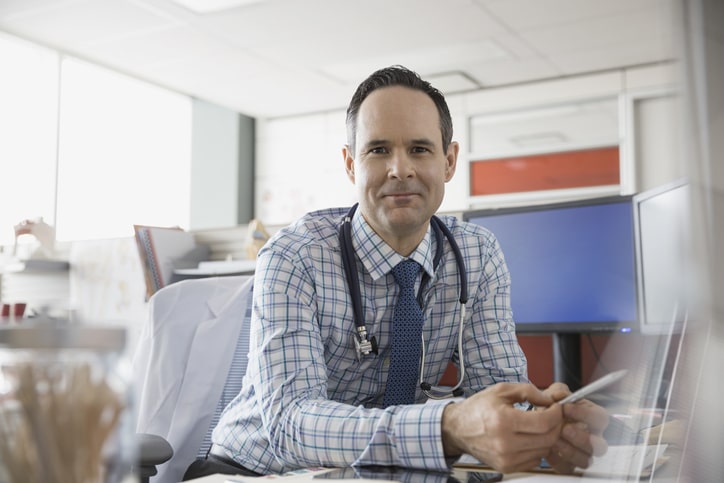
The Solution
Focus Patient found the right combination in Wordstat. They were able to implement their complete and extensive (thousands of items) ontology. The software hosts all the rules/algorithms necessary for their language processing requirements. They use the interactive version of the software to iteratively fine-tune the dictionaries, and then use the SDK, embedded in home-written Visual Studio programs to perform fast production analysis runs with each new batch of comments they collect.
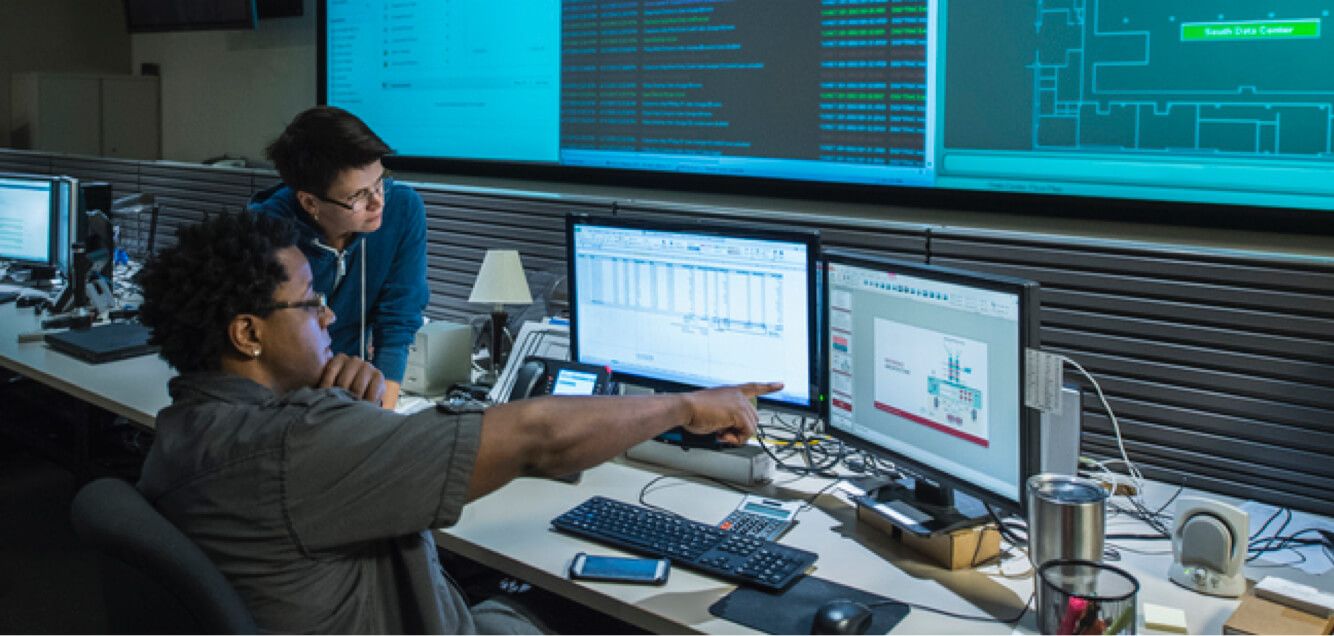
The Results
Once Focus Patient experts had experimented and documented the right sequence of tasks (from an Excel repository to an interactive version of a dictionary, then to a batch/SDK version), they established a production flow that is lean, reliable and fast, so that the whole stage between collecting testimonies and turning them into thematic sets is fluid, with all of this running on ‘power user’ type PCs.
They saved time in production, being able to close a monthly cycle (from raw texts to semantic analysis results stored in our database) typically in days.
The quicker turnover and new analysis/KPIs allowed them to attract new clients.
They enhanced their product offering by developing new and innovative business-relevant analysis (e.g. assessment of patients’ knowledge of their pathology) and KPIs (e.g. predictive non-adherence rate of a drug