IMPROVE SATISFACTION WITH AUTOMATED CUSTOMER VERBATIMS CLASSIFICATION
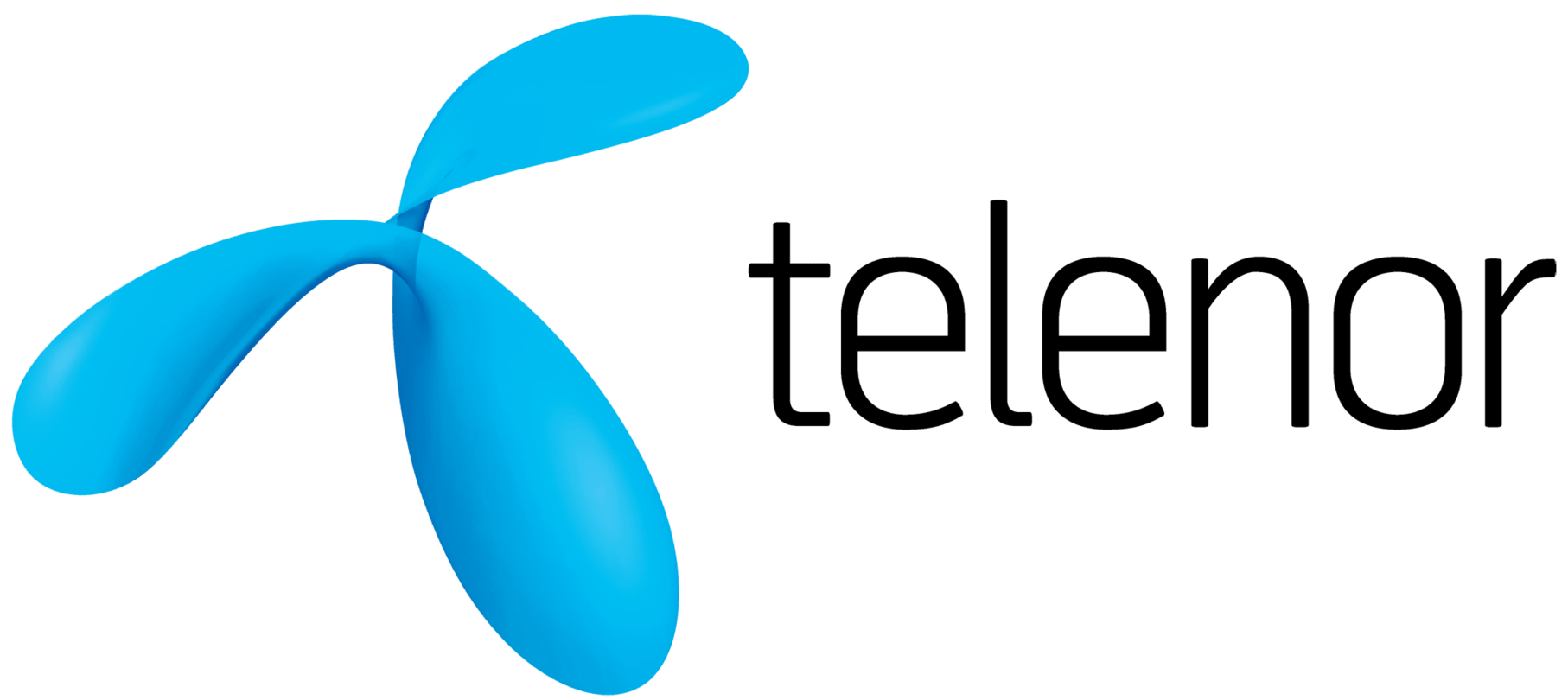
About the company
The Telenor group is a leading telecommunications company serving the Nordic countries and Asia. It has more than 182 million customers and annual sales of more than $US 13 billion. Telenor is #1 or #2 in most of its markets which include mobile operations in Norway, Sweden, Denmark, Finland, Pakistan, Bangladesh, Thailand, Malaysia, and Myanmar. The Telenor Group employs more than 20,000 people worldwide.
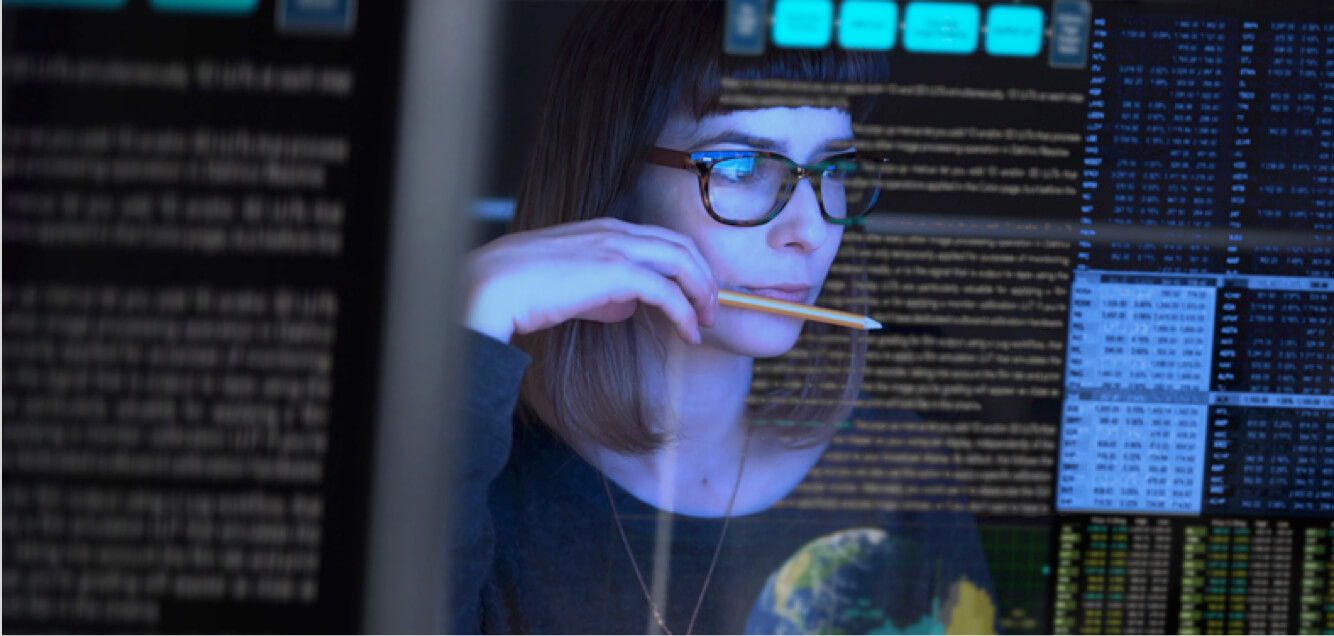
The Challenge
To quickly organize and manage thousands of text comments to improve efficiency and better understand what customers are saying. A customer service team in Telenor’s Norwegian business approached Jarl Moss Hildrum, Senior Research Scientist, AI, and Analytics team. Jarl’s team analyzes HR and customer data to see how they can improve decision making, streamline processes, make the business more efficient and responsive to its stakeholders.
The customer service team wanted to find a way to automatically categorize written messages (SMS) sent to Telenor by its customers. The business unit receives thousands of text messages from customers who contacted a call center. Organizing the content of these messages is important to be able to tell management what customers are saying so they can consider this in their decision making. The messages were being processed manually which was time-consuming, boring, and error-prone.
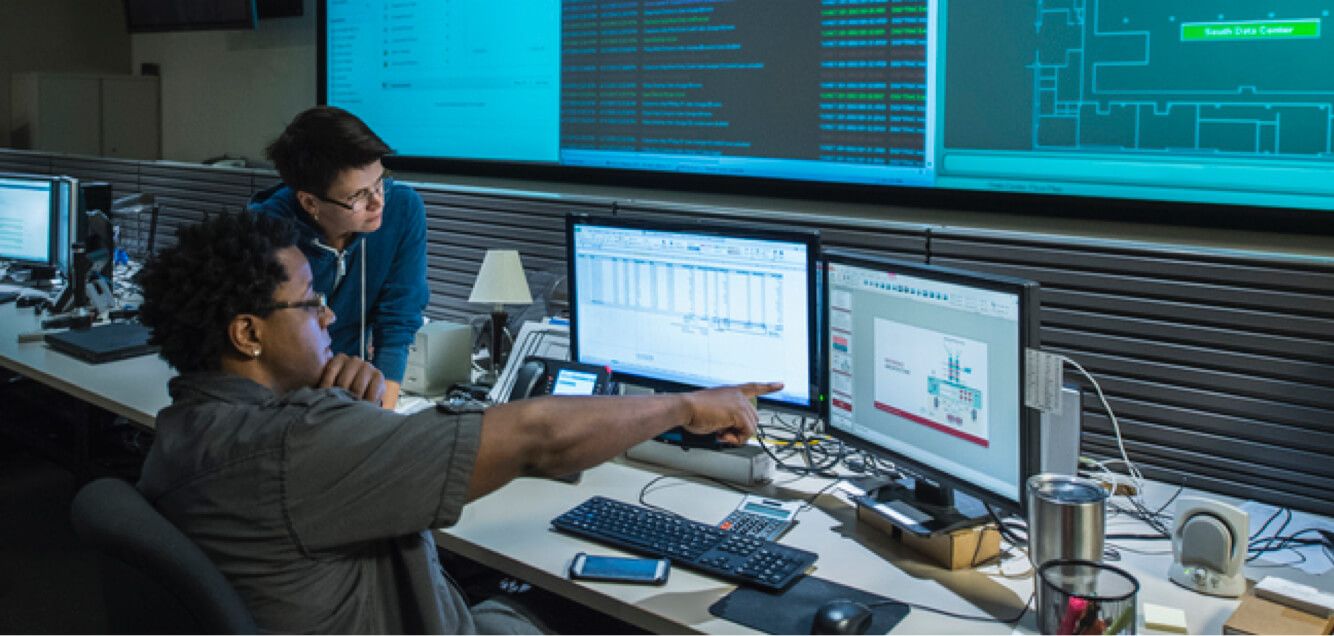
The Solution
Jarle and his team used the supervised machine-learning feature in WordStat to develop an automatic classifier. They uploaded a set of manually classified text messages to WordStat. The dataset consisted of sms-text messages that had been categorized into four different classes: customer service, customer agent behavior, policy, products, and services. This data was used to train a classifier to automatically classify new data into the same four classes. The classifier should be able to reach 90% accuracy or higher as it works with additional training data.
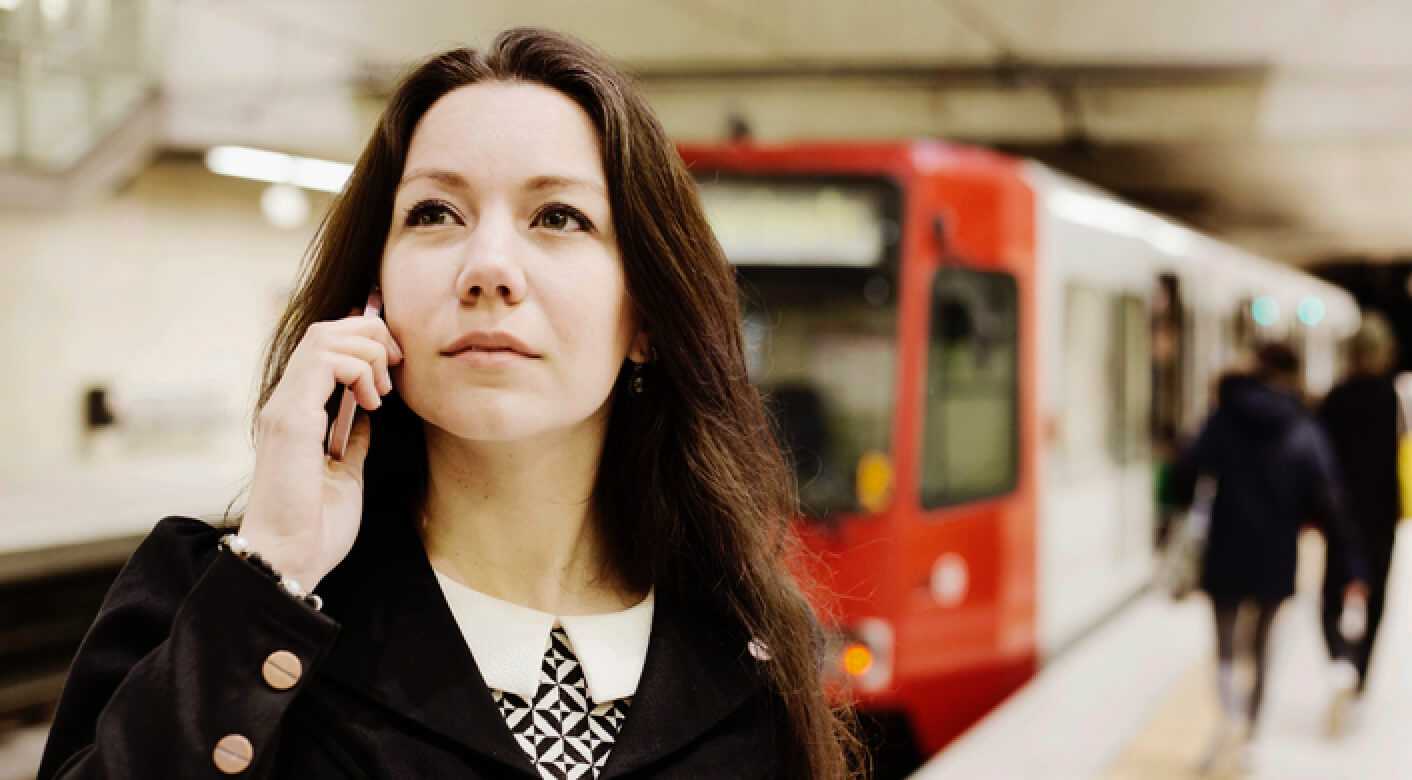
The Results
The classifier was useful to the customer service unit in several ways. With the automatic classifier in place, the analysts do not have to spend so much time doing manual classification. They are more motivated and can spend time doing other useful things. A quick back-of-the- envelope calculation shows an employee in the customer service unit spends about 8 hours manually classifying 1000 sms. The automatic classifier does the job in seconds.
Previously, manual classification was done about every second week taking about 16 hours altogether. This means a possible monthly salary savings of about $US 3,000 for one business unit. Across Telenor’s 8 business units, there is a potential of more than $US 20,000 savings per month. Using manual classification, the customer service unit could typically classified about 15% of the feedback. With automatic classification, all the feedback can be classified and reported to management in a condensed way. This means management receives more frequent and consistent information.